View the recordings of all the lectures on our YouTube Playlist, or select a specific presentation below.
About
Dates: March 17 – March 20 (2019)
Location: University of South Carolina
Topic: Models and Data
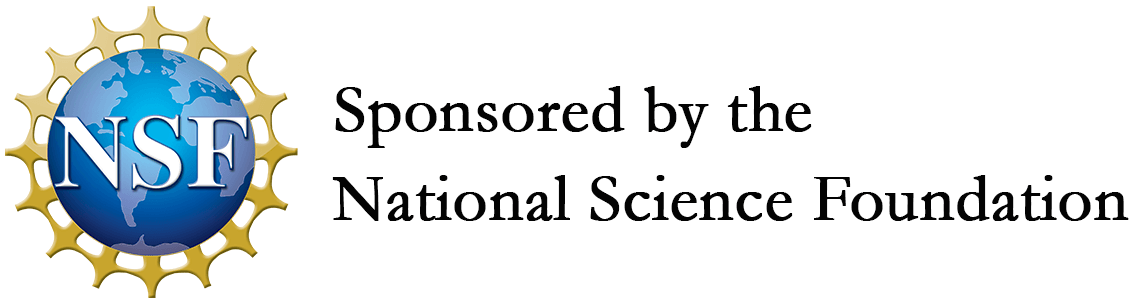
The 2019 Spring School has been funded by the National Science Foundation to support
all participants. It features five lecturers who are considered high-caliber representatives
of their respective areas of expertise. The lectures are tutorial in nature, interactive,
and are interlaced with open, group discussions.
The interactive lectures cover a diversity of topics organized under our main them,
namely to foster synergetic synthesis of on the one hand classical "model-based" and,
on the other hand "data-driven" methodologies. The topics addressed in the Spring
Schools pertain to ongoing vibrant developments in areas like machine learning, notable
deep learning, computational harmonic analysis, as a pillar of data science, or uncertainty
quantification and related modelling or numerical analysis aspects.
The topics concern several relevant areas in Data Science which is an emerging transdisciplinary
scientific field or research. The mathematical concepts treated in lectures and discussions
are therefore relevant for a large range of disciplines where data in combination
with physical/mathematical models provide major sources of information.
Invited Speakers
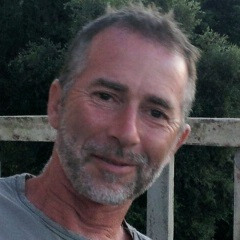
Albert Cohen
Least-Squares Methods for High Dimensional Problems
Abstract: Various mathematical problems are challenged by the fact they involve functions of
a very large number of variables. Such problems arise naturally in learning theory,
partial differential equations or numerical models depending on parametric or stochastic
variables. They typically result in numerical difficulties due to the so-called "curse
of dimensionality". We shall first discuss how these difficulties may be theoretically
handled in the context of stochastic-parametric PDE’s through the concept of sparse
polynomial approximation. We shall then focus on a class of concrete algorithms based
on least-squares fitting that provably achieve the convergence properties of these
approximations.
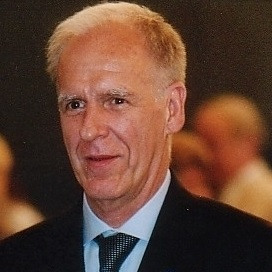
Ronald DeVore
An Overview of Approximation of Functions of Many Variables
Abstract: We discuss Approximation in High Dimensions, focusing on entropy widths, optimal recovery,
model classes, etc. We will round out the discussion with polynomial approximation
in high dimensions and neural networks, which is only peripherally high dimensional.
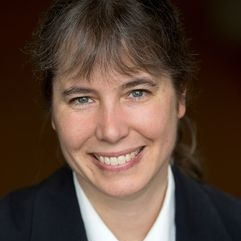
Gitta Kutyniok
Theory of Deep Learning
Abstract: Dr. Kutyniok’s main research topics include applied harmonic analysis, compressed
sensing, data science, deep learning, frame theory, high dimensional data analysis,
imaging science, inverse problems, machine learning, numerical analysis of partial
differential equations, sparse approximation.
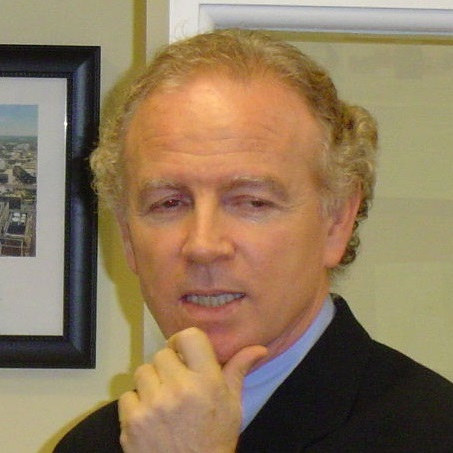
Eitan Tadmore
Collective Dynamics: Emergent Behavior with Long-Range and Short-Range Interactions
Abstract: Collective dynamics is driven by alignment that tend to self-organize the crowd, and
by different external forces that keep the crowd together. Prototype models based
on environmental averaging are found in opinion dynamics of human networks, self-organization
of biological organisms, and rendezvous of mobile systems. Different emerging equilibria
are self-organized into parties, flocks, tissues, etc. I will overview recent results
of collective dynamics driven by different "rules of engagement". I begin with a survey
of several classical models of agent-based systems, and follow with two fundamental
questions that arise in the context of such systems, namely --- their large time behavior
and their large crowd dynamics. In particular, I address the question how short-range
interactions lead, over time, to the emergence of long-range patterns, comparing geometric
vs. topological interactions. I conclude with a general framework which describes
the competition between pairwise alignment with external forcing.
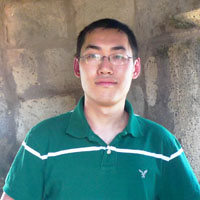
Changui Tan
Asymptotic Preserving Schemes on Kinetic Models with Singular Limits
Abstract: We will discuss kinetic models with singular hydrodynamic limits. The asymptotic
preserving (AP) schemes aim to provide a universal solver for both the full system
and the limit system, in the sense that the stability does not depend on the parameter.
For systems that have mono-kinetic singular limits, standard AP schemes lose accuracy
when the parameter is close to the limit. To overcome such difficulty, we introduce
a velocity scaling method that transforms the singular limit to a non-singular one,
and build AP schemes on the transformed systems.
Qingguang Guan
Poor Global Optima for Fully Connected Deep ReLU Neural Networks - Special Examples
for HD Approximation
Majid Noroozi
Clustering in Popularity Adjusted Stochastic Block Model
Simon Brugiapaglia
Compressive Sensing Approaches for High-Dimensional Function Approximation
Victor DiCaria
An Artificial Compression Based Reduced Order Model