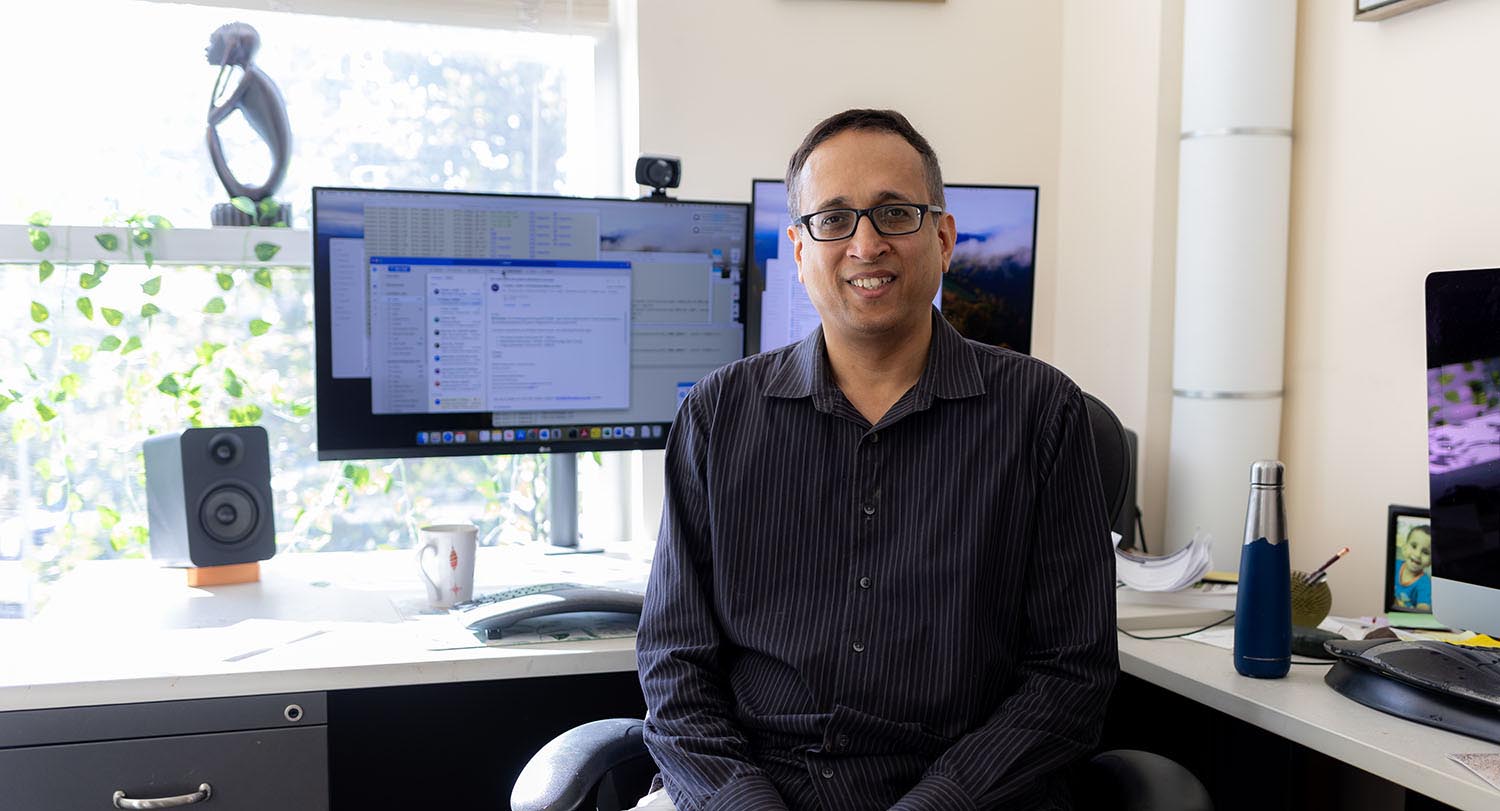
Artificial Intelligence advocates might defensively suggest, in good humor, that chatbots are “only human” and therefore prone to occasional mistakes. New research by a team at the University of South Carolina Department of Psychology basically confirms that notion with some important caveats.
“Even though the chatbots can be very powerful at solving complex problems, they can fail at relatively simple tasks that even children can do,” psychology professor Rutvik Desai says. For research purposes, the chatbots are referred to as “large language models,” or LLMs. “The models have become so good that people think they are as good as a human expert, but some of the problems they have is the tendency to make up stuff and put it forth confidently as fact, even though it is actually incorrect.”
Desai, along with postdoctoral fellow Nicholas Riccardi and graduate research assistant Xuan Yang, in September published their latest research, titled “The Two-Word Test as a Semantic Benchmark for Large Language Models” in the international peer-reviewed Nature Portfolio journal Scientific Reports.
Even though the chatbots can be very powerful at solving complex problems, they can fail at relatively simple tasks that even children can do.
The team of researchers developed a new, open-source benchmark called the Two-Word Test (TWT) to assess the semantic abilities of LLMs using 1,768 two-word, noun-noun combinations that only make sense semantically in a certain order. For example, “beach ball” versus “ball beach.” In separate exercises, a sample of humans and a selection of LLMs (GPT-4-turbo, GPT-3.5-turbo, Claude-3-Optus, and Gemini-1-Pro-001) were asked to distinguish meaningful word combinations and nonsense combinations.
“Results demonstrated that, compared to humans, all chatbot models performed poorly at rating meaningfulness of the phrases,” the researchers wrote in Nature’s Scientific Reports. The chatbots seemed to provide incorrect answers with great confidence.
Chatbots are trained to predict the next word, not unlike a super-advanced form of autocorrect, one of the earliest forms of AI that evolved from an algorithm developed from Microsoft Word’s glossary tool in the 1990s.
“The models don’t get a lot of examples of things that don’t make sense. They try to make sense of everything,” Desai says. “We ask ourselves, why is this failing when it is succeeding at so many other things, like writing poetry in the form of Shakespeare!”
Does not compute?
Riccardi notes that chatbots tend to get bewildered when presented with nonsensical TWTs because they presume all inputs are logical.
“The chatbot gets confused if you put two words together that are related, such as ‘ball, beach.’ It knows that the words are connected and happen together in some context,” he explains. “A TWT of ‘meat, kangaroo’ makes very little sense to a human, but the LLM will say a kangaroo has meat, so it’s logical to put them together.” They think/believe they can make sense of it.
One challenge in this type of research is the rapid pace of LLM development. Before one study is completed, the next generations of chatbots emerge in the marketplace with even greater abilities. Sometimes the research cannot keep pace with the technology development.
“The pace is really fast,” Desai says. “The technology is so exciting that billions of dollars are being invested in it.” Upgraded versions are introduced at an astonishing rate.
Even though this study showed large differences between LLMs and people in TWT exercises, Riccardi believes the evolving potential of AI is indisputable.
“These are extremely powerful tools. I use them daily for work, such as editing documents or to help with computer problems,” he says. “But they sometimes give answers that sound realistic and useful but are factually incorrect. Our work is highlighting that weakness.”
Caveat emptor
Riccardi considers his everyday utilization of AI as low risk. He uses it as a starting point to get ideas or an ending point for shortening and editing. It serves him as a time-saving, productivity enhancer.
Our study is a drop in the bucket in the larger field of studies. Mistakes these models make and why they make them is just one aspect of the entire puzzle moving forward.
“Our study is a drop in the bucket in the larger field of studies. Mistakes these models make and why they make them is just one aspect of the entire puzzle moving forward,” Riccardi notes. “We hope it will add to the discussion.”
Knowledge is key when using AI, and this research does not dispute the merits of AI. It simply suggests that the user beware.
“This research will help make people realize the AI models will work well in some situations, but they are not necessarily at the human level,” Desai concludes. “People should be very, very cautious in trusting the models. We are helping people understand the limitations of these models and not get carried away.”
Banner image: Photo by Jack Allen.