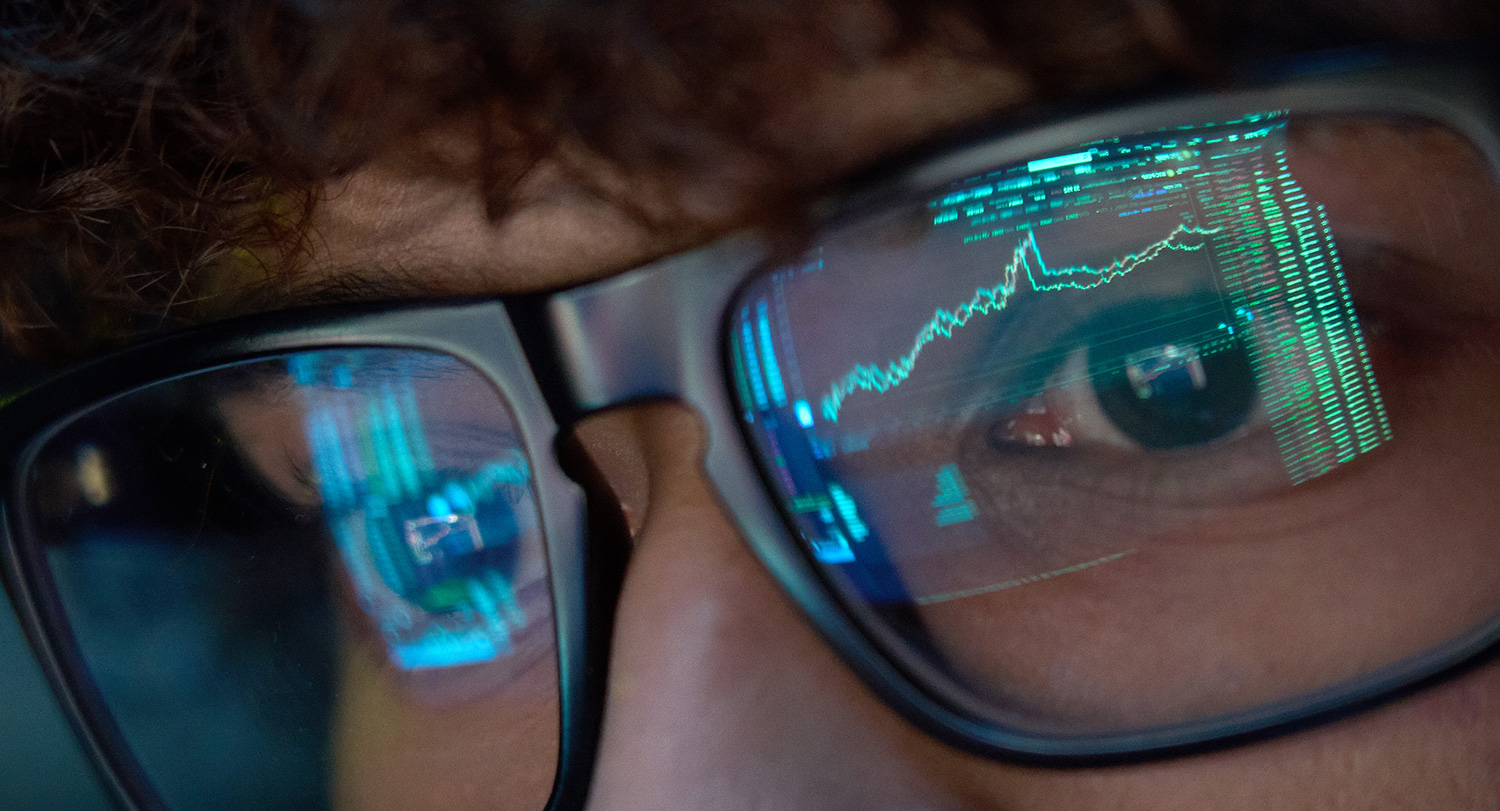
The human body is so complex that it’s estimated every person generates two terabytes of data every day.
If health care experts could gather and study that data, they could pinpoint ways for people and communities to be healthier.
The biggest problem — two terabytes per person is too much data.
“We’re not collecting one-hundredth of that data yet,” says Banky Olatosi, an associate professor in health services policy and management at USC’s Arnold School of Public Health. “If we’re able to find a way to collect that data, it will have a very big impact on being able to deal with your health,” he says, noting that it would lead to precise, personalized diagnoses.
Because data will play such a large role in the future of health care, the University of South Carolina launched the Big Data Health Science Center in 2019. The center held its fifth annual Big Data Health Science Conference in February, which attracted almost 100 presenters from five countries and 269 attendees. This was the first year the conference was partially sponsored by the National Institutes of Health.
“It shows how much the center and conference has grown to be nationally recognized,” Olatosi says of the NIH’s involvement.
Around 30 institutions were represented at the Columbia Metropolitan Convention Center, including universities, governmental organizations, industry and health care partners. “Year after year, our satisfaction surveys show attendees believe this conference is a great size in terms of not getting lost in your crowd,” says Big Data Health Science Center managing director Miranda Nixon.
“It’s small enough where you can have an individualized experience, but large enough where you can really network and form collaborations that you traditionally wouldn’t have,” she says.
Their common calling: to accelerate cutting-edge research and discovery.
Professor Xiaoming Li, who is the USC SmartState Endowed Chair for Clinical Translational Research, points to the variety of specific data types that were discussed.
“The conference covers artificial Intelligence and sensing, electronic health record data, social media data, genomic data, geospatial data,” Li says. “Also as part of the learning opportunities, we have student teams from across the nation, including our USC teams, compete over a 24-hour period to come up with analytical solutions to real data and real health issues they were given.”
Olatosi and Li are the co-leaders of the Big Data Health Science Center, and they know the challenges and opportunities on the horizon.
Here’s the big picture for what’s next for the Big Data Health Science Center and its supporters.
Big data in public health
Olatosi explains that big data can be used for disease management, prediction and treatment.
“COVID-19 was the clearest example of the use of big data for health care, for active surveillance to see what’s happening in real time, and to track the impacts of the virus across different geographical locations,” he says.
“Real time active surveillance is continuing to grow. It gives us the opportunity to intervene in people’s health care and their lives,” he says. “Data that’s been collected can be mined for interventions in the future.”
For the chronic diseases that afflict so many Americans such as diabetes or cardiovascular disease, big data can identify those who are most at risk, and then help tailor their lifestyle like nutrition and their diet and their food to improve their health.
We’ve already started seeing advances where based on your speech, we can predict whether you’re going to have cognitive decline in the future. And that’s a precursor to being able to then diagnose whether you’re going to be at risk for Alzheimer’s or other dementias.
Olatosi notes that despite advances in what data can now tell researchers, there still must be dialogue with those seeking care.
“Long COVID is the only disease condition in our lifetime that was discovered by patients,” he says. “They were the ones who said there’s something going on. The health community was like, ‘No, it’s all in your head.’”
Those patients then formed online support groups where they could share their symptoms and experiences.
“The academic community saw that and said, ‘Maybe there is something,’ and then it translated to find the scientific basis of it,” Olatosi says.
Collecting data, maintaining privacy
Big data analysis requires that researchers know where data is and the steps for retrieving it. First, they must get permission to access it in a way that ensures patient privacy. Next, they must identify whether the data is usable in the form it’s delivered. Then they’ll create a schedule for when the data will be updated.
Most data remains siloed among different data owners. That necessitates negotiations for access to data in order to see a fuller picture.
“Ideally, in the future, we’ll be able to get real-time data,” Olatosi says. “But we’re not at that point yet. Because data has to be cleaned and verified.”
A prime example is insurance claims data. “Every service that you receive in a health care facility has a billing code attached to it,” Olatosi says. Most groups will not release the data until as much as three to six months after the patient’s visit to allow for if there were mistakes in the billing or disputed claims.
“It’s messy,” Olatosi says. “All of it has to be reprocessed and goes back and forth, because if they give you that data in real time, it will have errors baked into it. And whatever you do with that data would also have errors.”
Data access remains a landscape that is not completely free of barriers. Researchers find themselves working to reduce those barriers while maintaining patient confidentiality and data security.
“It’s not helpful when we hear about data breaches and how that impacts trust in AI,” Olatosi says. “There are risks associated with accessing data.”
Predictors for a clearer picture
Researchers can now use neuroimaging, or MRI, to predict someone’s likelihood of having a future disease. Say you get a scan to detect for a specific symptom. In the past, that imaging data would be filed away and forgotten. Now, AI can learn from that image and can compare to other patients how an undetected disease condition is progressing.
“From that image, we can create an algorithm that once it sees your image, whether you’re at the beginning stage, or you’re in the middle stage, they can predict for you what your likelihood is of having this condition in the future, Olatosi says.
Embedded in Artificial Intelligence are large language models (LLMs), the most famous of which is ChatGPT. Big data researchers will be using ChatGPT and similar LLMs in a massive way. And the entry point will be chatbots and similar automated services.
“Most people don’t like to deal with automated services,” Olatosi says. “But those automated services are going to be very, very powerful going on in the future. Because they are going to be listening to your tone, they are going to be listening to your voice, they are going to detect whether you’re sad.”
With their large capacity to quickly process information, LLMs will be in a position to make prognoses.
“We’ve already started seeing advances where based on your speech, we can predict whether you’re going to have cognitive decline in the future,” Olatosi says, “and that’s a precursor to being able to then diagnose whether you’re going to be at risk for Alzheimer’s or other dementias.”
Directing AI skill sets toward health care
One of the biggest challenges in the way of realizing big data success in health care is workforce. Historically, researchers in AI and data science attracted the attention of the financial industry.
“We don’t have enough people in this area,” Olatosi says. “The people with the skill sets that we need are in high demand in other industries that pay way more than health care can afford.”
That’s why the center maintains workforce training pipeline programs. They start at the undergraduate level with training that targets their development in the hopes that they’ll want to continue to the master’s level. At the doctoral level, a program targets pre-doctoral training, and there is support for junior faculty as well.
There is also training for community scholars. “That is, talking to people from the community to learn about what this is, not be afraid of it and then become champions in their own community on the benefits of this,” Olatosi says.
“Without that workforce, you’re not going to be able to grow as quickly as you want in this area,” he says.
The dedication of USC’s students was realized when a team won the annual conference’s 24-hour student competition for the first time. Each school’s team was presented with real data to address analytical solutions for real health issues.
“They loved it. We were proud,” Olatosi says. “They’re very competitive. They don’t sleep well during the taxing competition, which is ironic because this year’s study was on sleep.”
The next Big Data Health Science Conference will be Feb. 13-14, 2025, when it moves to a Thursday-Friday time frame at the Pastides Alumni Center.
Olatosi is looking forward to how all the advances in research for technology, data analysis, patient engagement and workforce will be coming together.
“Eventually, we’re going to have the capacity to really get all the data occurring in you in real time,” he says. “And that’s going to be a game-changer for precision medicine.”