Department Highlights
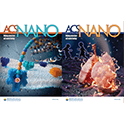
Liu publishes two papers in high impact journal
Chemical and Biomedical Engineering Associate Professor Chang Liu and his research group recently published two cover articles in the high impact journal, ACS Nano.
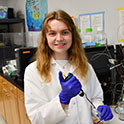
DePratter one of four USC students named Goldwater Scholars
Biomedical engineering student Shannon DePratter recently earned a national award that recognizes the outstanding work and research of STEM undergraduates.
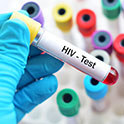
Bringing HIV testing to patients
Chemical and Biomedical Engineering Assistant Professor Chang Liu is currently working on research that aims to develop a specialized HIV self-test that could help lower transmission rates and allow for early treatment to reduce the chances of developing AIDS.
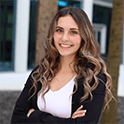
Student Spotlight: Lauren Speck
Senior Lauren Speck developed an interest in engineering when her grandmother was diagnosed with Parkinson's disease. Combined with her interests in science and math, Speck's personal experience caring for her grandmother ultimately led her to pursue a degree in biomedical engineering.
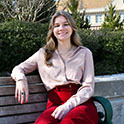
Sewing and Swearingen
Biomedical engineering junior Mina Schaafsma is quite a talented student. Whether it is doing research or making her own clothes, she always makes time to pursue her academic and creative interests.
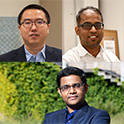
Chang Liu, one of three CEC 2023 USC Breakthrough Award winners
The Office of the Vice President for Research is pleased to announce the exceptional faculty members and graduate students selected to receive the 2023 Breakthrough research awards.